AI-Driven Predictive Analytics: Uncovering Trends and Making Informed Decisions
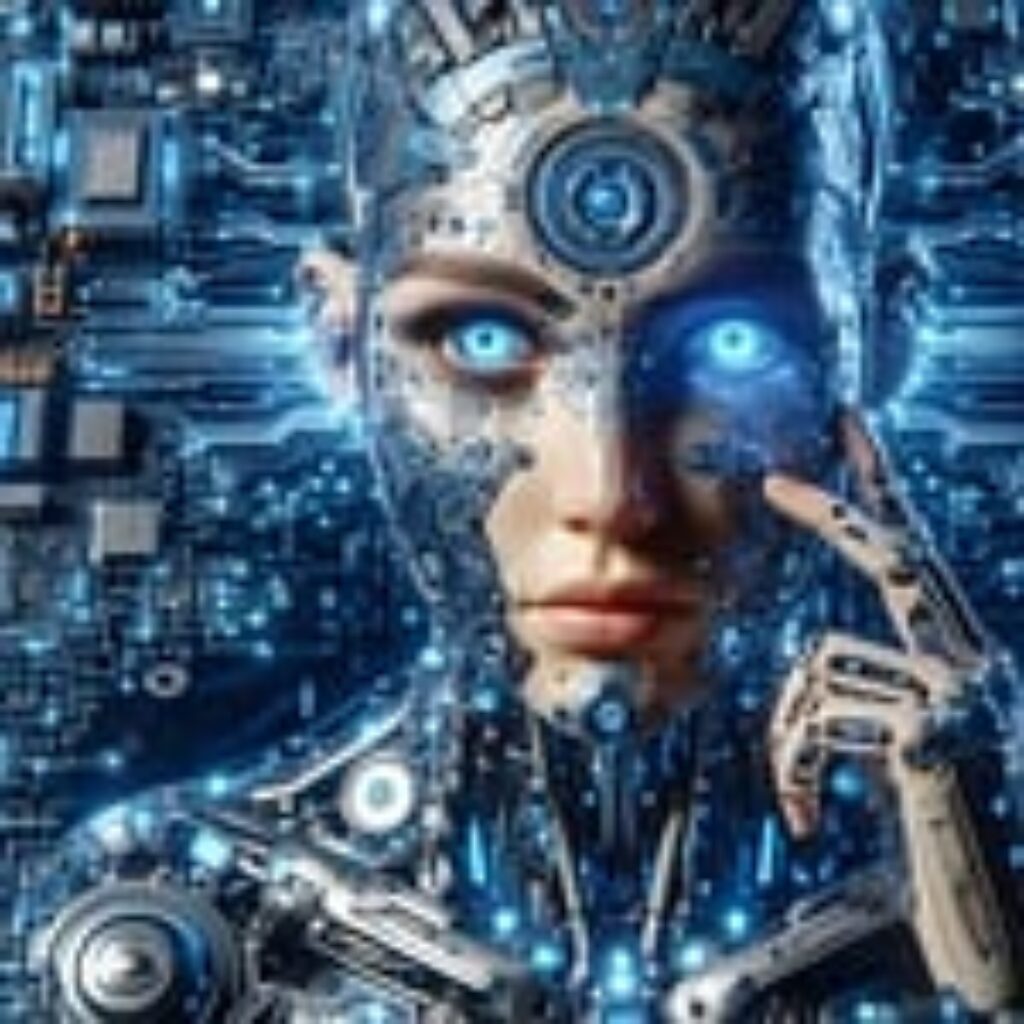
AI-Driven Predictive Analytics: Unveiling Trends and Empowering Informed Decisions
Predictive analytics is the use of data, statistical algorithms, and machine learning techniques to identify patterns and predict future outcomes. AI-driven Prognostic analytics takes this concept a step further by leveraging artificial intelligence (AI) algorithms to enhance the accuracy and efficiency of predictive models.
Key Technologies Behind Predictive Analytics
Key technologies used in predictive analytics include:
– Machine Learning Algorithms: Supervised and unsupervised learning algorithms learn from historical data to identify patterns and make predictions. Common algorithms include linear regression, decision trees, and neural networks.
– Data Mining Techniques: Data mining techniques are used to extract meaningful insights from large datasets. Techniques such as clustering, classification, and association rule mining are commonly employed.
– Statistical Modeling: Statistical models are used to represent the relationships between variables and predict outcomes. Techniques such as regression analysis, time series analysis, and Bayesian inference are widely used.

Applications in Various Industries
AI-driven Prognostic analytics has applications in numerous industries, including:
– Financial Services: Predicting customer churn, loan defaults, and market trends.
– Healthcare: Diagnosing diseases, predicting patient outcomes, and personalizing treatment plans.
– Retail: Forecasting demand, optimizing inventory levels, and providing personalized recommendations.
– Manufacturing: Predictive maintenance, optimizing production processes, and improving quality control.
Benefits of AI-Driven Prognostic analytics
– Improved Accuracy: AI algorithms can learn complex relationships and identify patterns that may be missed by traditional statistical methods, leading to more accurate predictions.
– Increased Efficiency: AI-driven Prognostic analytics automates the process of data analysis and model building, saving time and resources.
– Enhanced Insights: AI algorithms can uncover hidden insights and relationships in data, providing deeper understanding of underlying trends and patterns.
Challenges and Considerations
– Data Quality and Availability: The accuracy of predictive models depends on the quality and availability of data.
– Model Interpretability: Complex AI models can be difficult to interpret, making it challenging to understand the rationale behind predictions.
– Ethical Considerations: The use of AI for predictive analytics raises ethical concerns, such as bias, privacy, and discrimination.
Future Trends in Prognostic analytics
– Integration with IoT and Big Data: Predictive analytics will increasingly be integrated with IoT and big data to analyze real-time data and make more accurate predictions.
– Advanced AI Algorithms: The development of more advanced AI algorithms, such as deep learning and reinforcement learning, will further enhance the capabilities of predictive analytics.
– Democratization of Predictive Analytics: User-friendly tools and platforms will make predictive analytics more accessible to non-technical users.
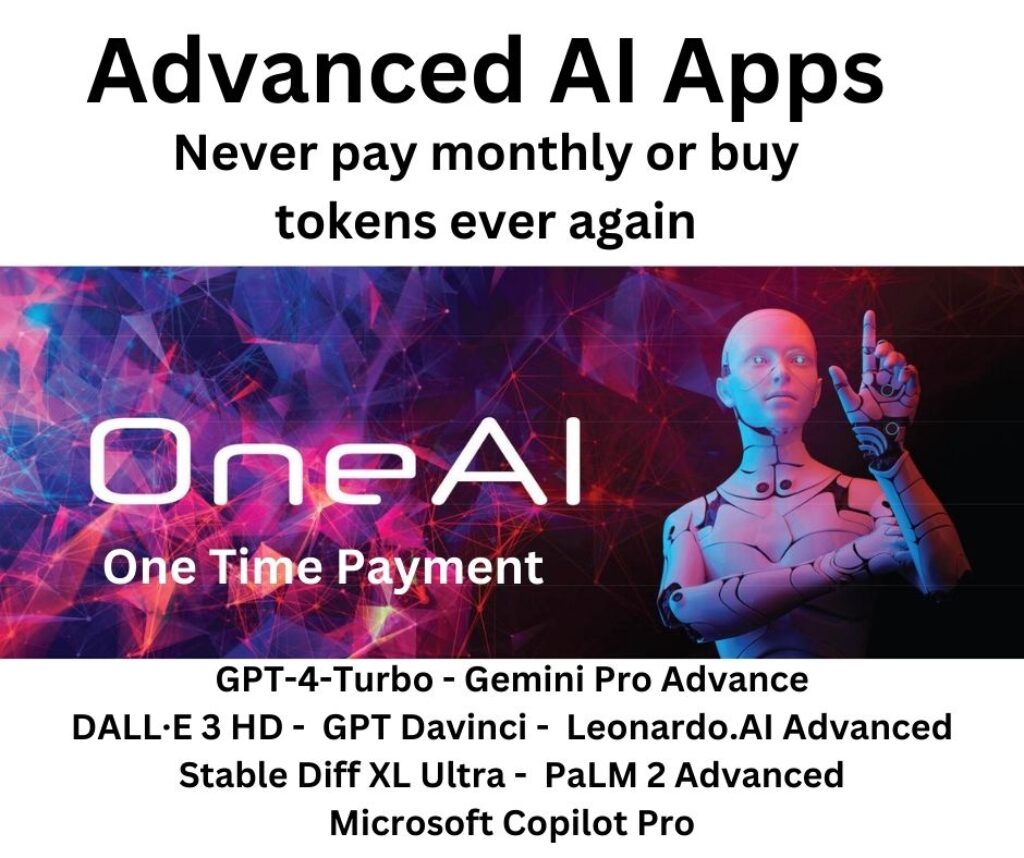